Blog Post
Artificial Intelligence and Machine Learning in Fleet – Driving the Future Ahead
By Parsh Wanath
April 3, 2025
Artificial Intelligence (AI) and Machine Learning (ML) are transforming industries, driving innovation, and arguably shaping up to be the most significant technological advancement since the internet. Many fleet professionals are asking: How will AI and ML impact fleet management?
In this blog, I’ll break down the key business impacts and opportunities that AI and ML present so you can strategically prepare for the future.
The Business Impact of AI in Fleet
AI is helping businesses become more productive. When implemented effectively, it enhances decision-making, streamlines processes, and drives innovation. Let’s explore the core benefits AI brings to fleet management.
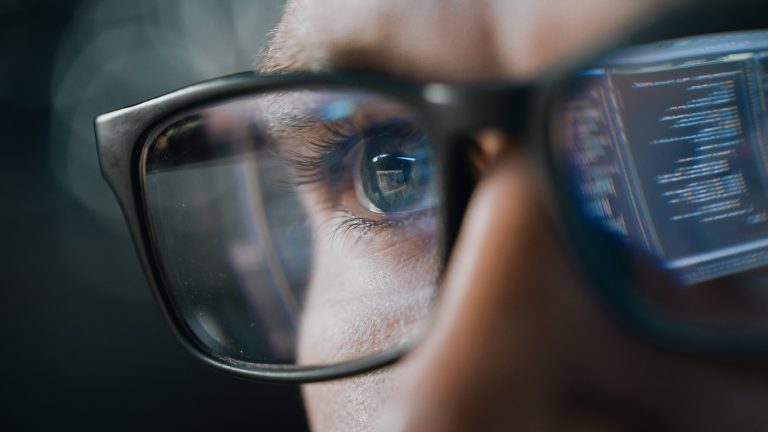
1. Empowering Business Leaders with Insights
AI/ML enables fleet leaders to make more informed strategic decisions. Companies leveraging AI gain a competitive edge through enhanced data-driven decision-making that goes beyond reactive measures to proactive strategies, ultimately leading to a more agile, cost-effective, and future-proof fleet operation.
2. Increasing Operational Efficiency
One of AI’s most immediate benefits is automation. Repetitive and time-consuming tasks where a human adds small value—such as data entry, simple decision making, and error detection—can be automated, allowing employees to focus on higher-value work. The result? Increased productivity, reduced re-work, and cost savings.
3. Driving Innovation and Unlocking New Opportunities
AI doesn’t just optimize existing processes—it sparks innovation. Focusing on operational efficiency can transform a process and result in competitive differentiation, however the possibility of AI to create new business models/products via dramatic alteration of core processes is exciting. However, this type of investment comes with substantial uncertainty — i.e. long-term strategic bets that must be planned as return on future instead of the usual return on investment (ROI).
4. Enhancing Customer Satisfaction
High quality products and efficient fleet management solutions, enhanced by AI’s ability to personalize customer interactions, anticipate client needs, and streamline communication will lead to increased customer satisfaction and loyalty.
How AI is Helping Manage Big Data
In today’s digital landscape, companies grapple with managing data at scale and the challenge of finding actionable insights within this “big data” is akin to searching for a data needle in a vast digital haystack. The new generation of fleet professionals, as digital natives, inherently expect instant access to information–a behavior deeply ingrained through daily interactions such as searching Google for answers or asking Siri for immediate assistance. This expectation for instant information access will fundamentally alter the way fleet professionals interact with the fleet management systems and necessitates a shift in how information is delivered and consumed.
How AI Helps Unlock Value from Data
Agentic AI, a form of artificial intelligence that operates independently, performing tasks and making decisions without human intervention, is emerging alongside digital assistants as powerful solutions for navigating this “digital haystack.” These intelligent agents can autonomously sift through large, complex, and highly diverse fleet data, proactively identifying specific insights that human analysts might overlook, and act. With the right AI solutions, organizations can:
- Extract meaningful insights faster and more accurately.
- Improve response times through smart automation.
- Enable seamless access to data via multiple channels, including mobile apps, web portals and AI-assisted customer support.
The Power of Machine Learning
While the emergence of generative AI tools like Chat GPT have captured significant attention from the zeitgeist, it’s crucial not to overlook the enduring importance of traditional machine learning. These established techniques are fundamental for tasks like classification, regression, and anomaly detection, providing critical capabilities for understanding existing data patterns and making predictions. Traditional machine learning excels at analyzing structured data to drive informed decision-making, optimize processes, and automate specific tasks with high accuracy. For fleet management, this means:
- Predictive Maintenance – Identifying potential vehicle failures before they happen
- Fuel Fraud Detection – Analyzing data to identify potential fraudulent fuel transactions
- Conversational Analytics – Extracting insights from natural language conversations between customers and service and support channels
- Fuel Optimization – Analyzing driving patterns to reduce fuel consumption
- Dynamic Route Planning – Adjusting routes in real-time based on traffic and weather conditions
As Machine Learning models iteratively learn from more data, they continuously refine their accuracy over time, leading to smarter, more efficient fleet operations.
The AI Challenge: Security and Privacy Considerations
While AI offers transformative potential for businesses, a critical challenge lies in carefully navigating the complex landscape of data security and privacy. Companies must proactively address these concerns to foster trust and ensure responsible AI adoption. Fleet management companies are custodians of their clients’ data. As good data stewards, striking a balance between leveraging the power of AI for optimized fleet performance and rigorously protecting sensitive data is not merely a best practice, but an essential prerequisite for sustainable and ethical AI implementation.
Data Privacy
Prioritizing data privacy is paramount for safeguarding individuals’ personal information from misuse and harm, fostering trust with customers and stakeholders, and ensuring compliance. Here are a few considerations:
- Take robust measures to ensure that proprietary or Personally Identifiable Information (PII) data is not exposed to public AI models.
- When building/training proprietary AI models ensure the data is anonymized.
- Consider building retrieval – Augmented Generation (RAG) AI models, which are designed with inherent data privacy safeguards. The data remains entirely separate and is exclusively utilized to provide relevant context during the AI generation process, ensuring that sensitive information is not integrated into the model itself, and its use is strictly controlled.
Data Security
Implementing various measures to protect information from unauthorized access, misuse, and loss is equally relevant in AI as well. Here are a few considerations:
- Meticulous Data Source Selection – Use only curated, reliable, and secure data sources for AI training and analysis.
- Data Minimization – Restrict data access strictly to what is absolutely necessary for the specific AI application.
- Robust Access Control Policies – Guarantee that only authorized users are permitted to interact with sensitive information, thereby mitigating the risk of unauthorized access or data breaches.
The Future Ahead
Artificial Intelligence and Machine Learning are not just emerging technologies—they are redefining the way businesses operate. AI/ML is poised to transform fleet management by enhancing efficiency, driving innovation, and improving customer experiences.
For fleet professionals, the question is no longer if AI/ML will make an impact but how you will leverage it to stay competitive.
If you have questions about how AI can transform your fleet operations, reach out to us at wheels.com—we’re here to help you navigate the road ahead.
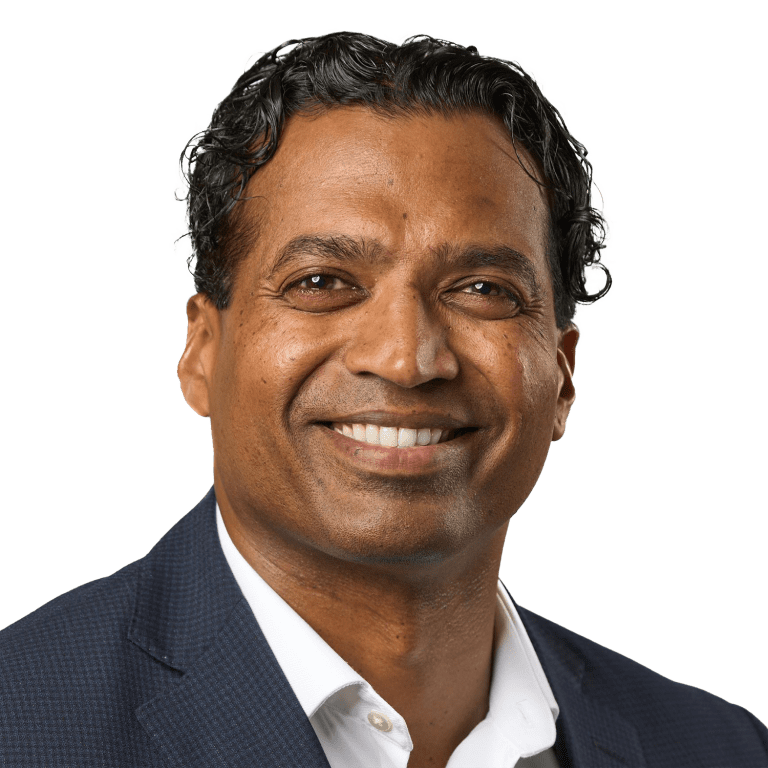
VP Digital Engineering and Advanced Analytics
Originally published by AFLA Fleet Insights.